Small language models have become increasingly popular in business operations. They are a type of natural language processing (NLP) model that can be trained on a small amount of data. Small language models are designed to be more efficient and cost-effective than larger models, making them an attractive option for businesses looking to implement NLP technology.
The potential strategic advantages of small language models in business are numerous. They can be used to automate customer service chatbots, analyze customer feedback, and improve search engine optimization. Small language models can also be used to analyze data from social media and other sources to gain insights into customer behavior and preferences.
Key Takeaways
- Small language models are a cost-effective and efficient option for businesses looking to implement NLP technology.
- Small language models can be used to automate customer service chatbots, analyze customer feedback, and improve search engine optimization.
- Small language models can provide valuable insights into customer behavior and preferences by analyzing data from social media and other sources.
Strategic Advantages of Small Language Models
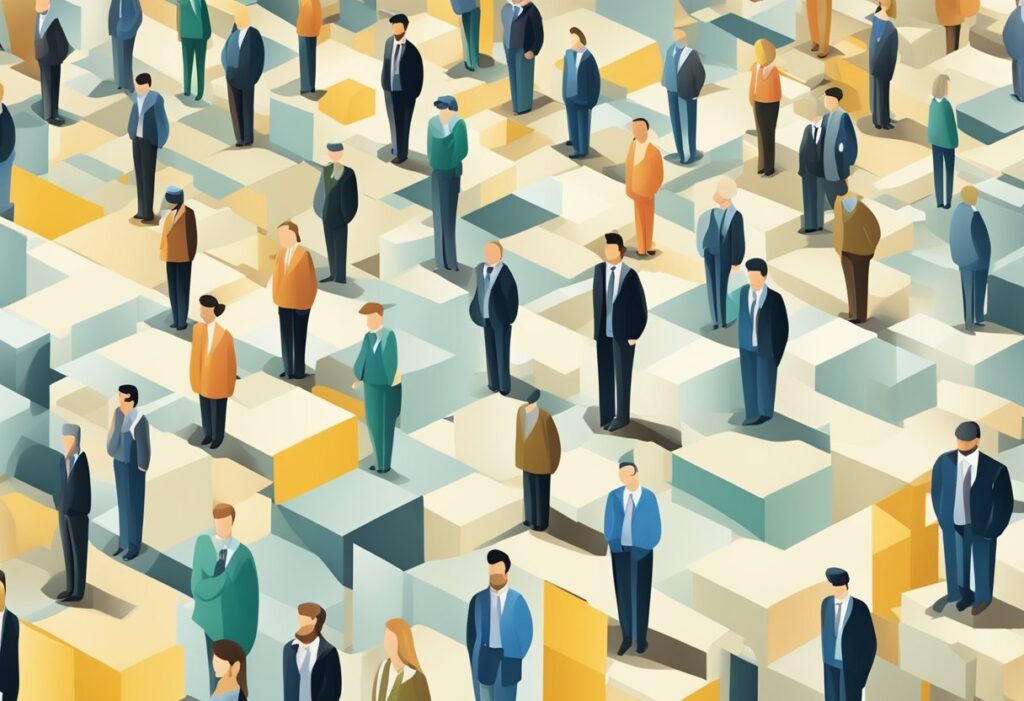
Small language models have been gaining popularity in recent years due to their strategic advantages over larger models. In this section, we will discuss the key strategic advantages of small language models in business.
Cost Efficiency
Small language models are more cost-efficient than their larger counterparts. They require less computational power and memory, which translates to lower hardware and maintenance costs. This makes them an ideal choice for small and medium-sized businesses with limited budgets. Additionally, smaller models require less data for training, which further reduces the cost of building and maintaining language models.
Ease of Integration
Small language models are easier to integrate into existing systems and workflows. They can be deployed faster and with less disruption to existing processes. This makes them an ideal choice for businesses that want to implement language models quickly and with minimal overhead. Smaller models can also be used to augment existing systems, such as chatbots and virtual assistants, to improve their accuracy and effectiveness.
Customization Potential
Small language models are more customizable than larger models. They can be trained on specific domains, such as legal or medical, to improve their accuracy and relevance. This makes them an ideal choice for businesses that need language models tailored to their specific needs. Additionally, smaller models can be fine-tuned with less data, which makes it easier to customize them for specific use cases.
In summary, small language models offer a cost-efficient, easy-to-integrate, and customizable solution for businesses looking to implement language models. By leveraging these strategic advantages, businesses can improve their workflows, customer experiences, and decision-making processes.
Applications in Business Operations
Small language models have the potential to revolutionize the way businesses operate. They can be used for a variety of tasks, including customer service enhancement, market analysis, and automated reporting.
Customer Service Enhancement
Small language models can be used to enhance customer service by providing personalized responses to customer inquiries. By analyzing customer data, a small language model can provide recommendations and solutions to customers based on their individual needs and preferences. This can lead to increased customer satisfaction and loyalty.
Market Analysis
Small language models can be used to analyze market trends and consumer behavior. By analyzing data from social media and other sources, a small language model can identify patterns and trends that can help businesses make informed decisions about product development, marketing strategies, and customer engagement.
Automated Reporting
Small language models can also be used to automate reporting tasks, such as generating financial reports and sales forecasts. By analyzing data from various sources, a small language model can generate reports that are accurate, concise, and easy to understand. This can save businesses time and resources, allowing them to focus on other important tasks.
Overall, the potential applications of small language models in business operations are vast and varied. As the technology continues to evolve, businesses are likely to find new and innovative ways to use these models to improve their operations and stay ahead of the competition.
Challenges and Limitations
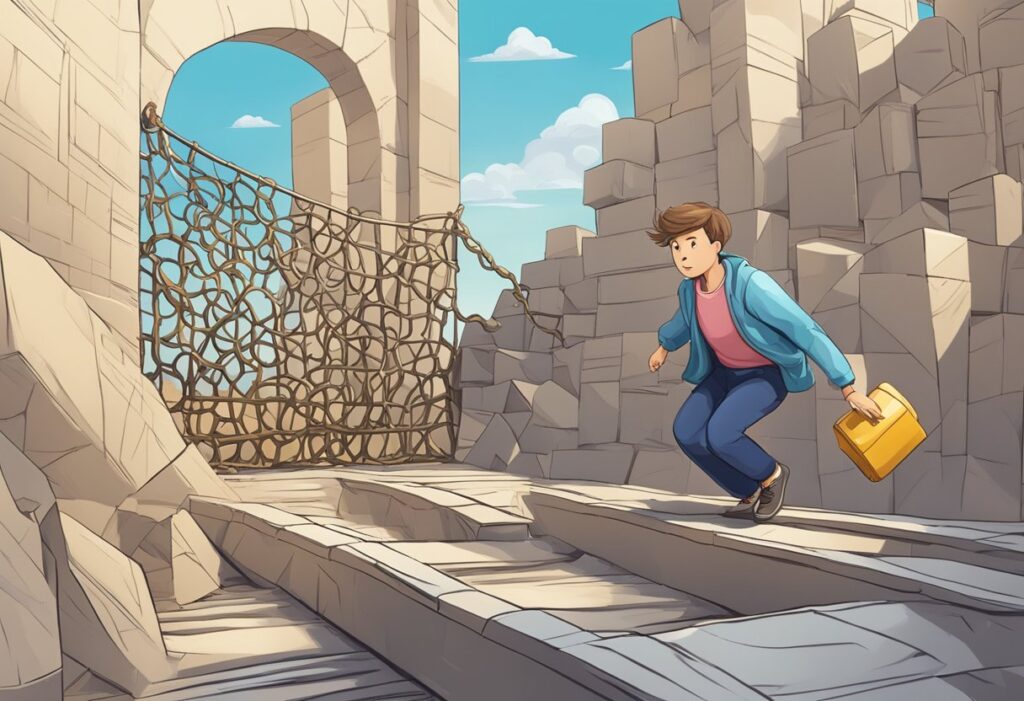
Small language models are a promising technology that can provide businesses with cost-effective solutions for natural language processing tasks. However, there are several challenges and limitations that must be considered before implementing them. This section discusses some of the most significant challenges and limitations of small language models.
Data Privacy Concerns
One of the most significant challenges of small language models is data privacy concerns. Small language models are usually trained on large datasets that contain sensitive information. Businesses must ensure that the data they use to train their models is secure and that the models themselves do not leak any sensitive information. This requires businesses to implement robust data security measures and to ensure that their models comply with data privacy regulations.
Scalability Issues
Another major challenge of small language models is scalability. Small language models may not be suitable for large-scale natural language processing tasks, as they may not have the capacity to process large amounts of data. This can limit the usefulness of small language models for businesses that require large-scale natural language processing solutions. To address this challenge, businesses may need to consider using larger language models or other natural language processing solutions that are better suited to their needs.
Accuracy and Reliability
Small language models may also have accuracy and reliability issues. Due to their limited capacity, small language models may not be able to accurately process complex language patterns or generate coherent responses to complex queries. This can limit the usefulness of small language models for businesses that require accurate and reliable natural language processing solutions. To address this challenge, businesses may need to consider using larger language models or other natural language processing solutions that are better suited to their needs.
In conclusion, small language models have great potential for businesses looking for cost-effective natural language processing solutions. However, businesses must be aware of the challenges and limitations of small language models before implementing them. By addressing these challenges, businesses can ensure that they are using the right natural language processing solutions for their needs.
Future Outlook
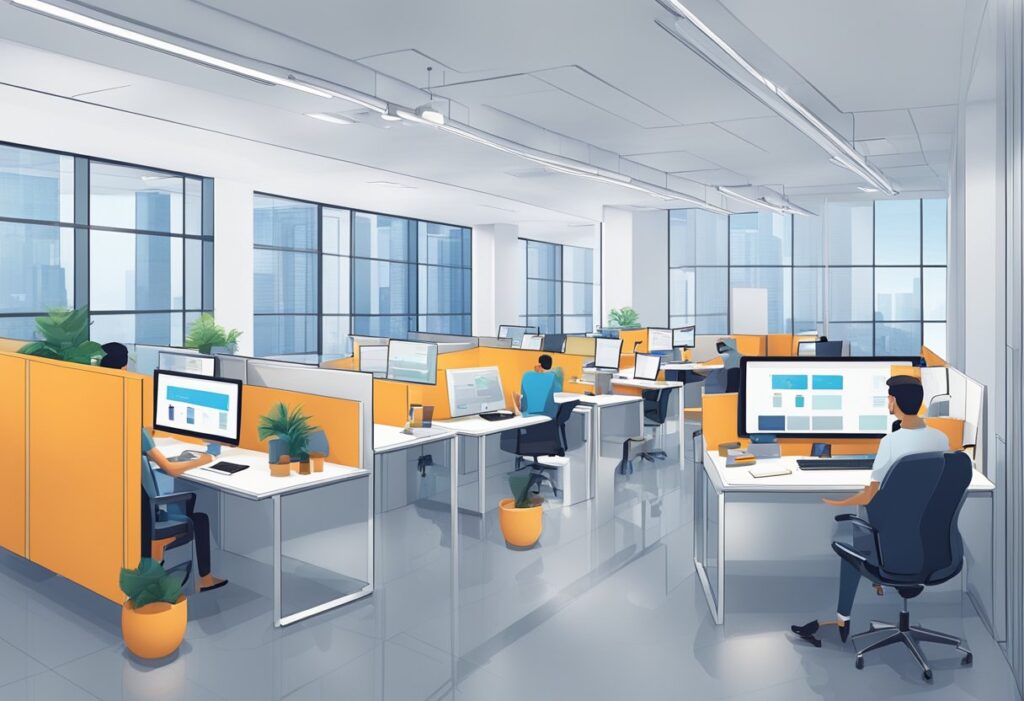
Technological Advancements
Small language models have shown promising results in various business applications, and their potential is likely to grow in the future. With the advancements in technology, small language models are expected to become more efficient and effective in performing complex tasks. The integration of machine learning and artificial intelligence in small language models will further enhance their capabilities, making them more accurate and reliable.
One of the most significant technological advancements in small language models is the development of transformer-based models. These models have shown remarkable performance in natural language processing tasks and have become the standard for many language models. The transformer architecture has also paved the way for the development of more efficient and effective small language models, which can perform complex tasks with greater accuracy and speed.
Industry Adoption Trends
The adoption of small language models in the business sector is expected to rise significantly in the coming years. The use of small language models can help businesses automate various tasks, improve customer experience, and gain insights into customer behavior.
Small language models can be used in various industries, including healthcare, finance, retail, and e-commerce. In the healthcare industry, small language models can be used to analyze patient data, improve diagnosis accuracy, and develop personalized treatment plans. In the finance industry, small language models can be used to analyze financial data, detect fraud, and make investment decisions. In the retail and e-commerce industry, small language models can be used to improve customer experience, develop personalized marketing strategies, and analyze customer feedback.
Overall, the future outlook for small language models in the business sector is promising. With technological advancements and industry adoption trends, small language models are expected to become more efficient, effective, and widely adopted in various business applications.
Best Practices for Implementation
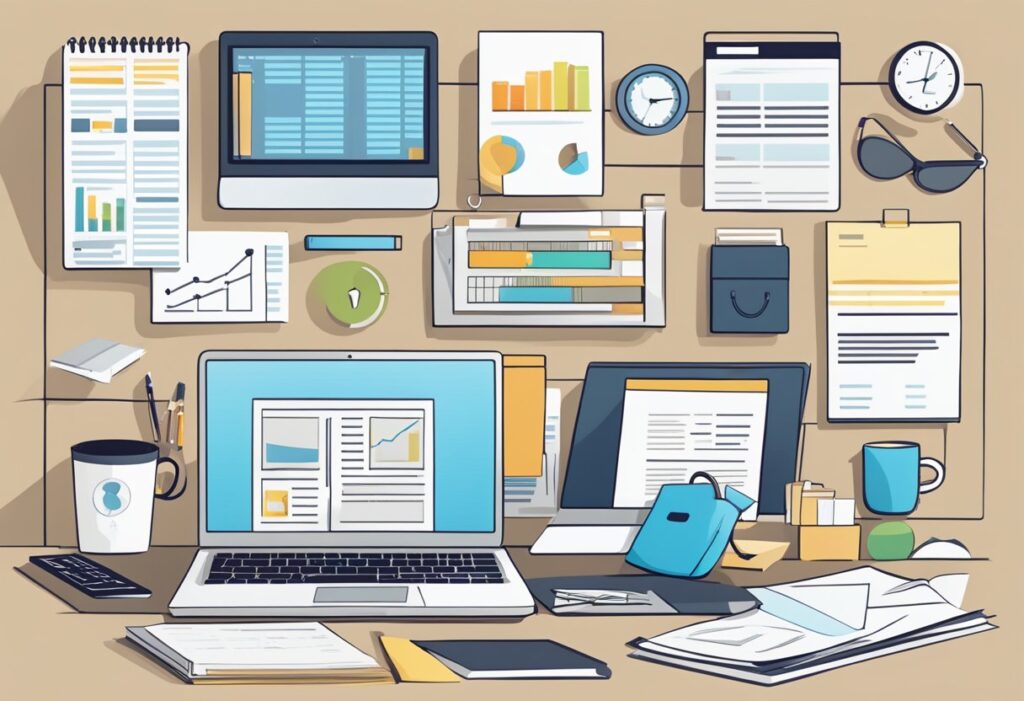
Choosing the Right Model
Choosing the right language model is a crucial step in implementing small language models in business. It is important to select a model that is appropriate for the specific use case and the amount of data available. Some popular models for small businesses include GPT-4, Microsoft Copilot, and Gemini. These models have shown to be effective in various natural language processing tasks such as sentiment analysis, text classification, and language translation.
Continuous Training and Updates
Small language models need to be continuously trained and updated to ensure their accuracy and relevance. This involves feeding the model with new data and retraining it periodically to improve its performance. It is also important to keep the model up to date with the latest advancements in natural language processing techniques and tools. This can be achieved by following relevant research papers and attending conferences and workshops.
Measuring Impact and ROI
Measuring the impact and return on investment (ROI) of small language models is essential to determine their effectiveness and justify their implementation. This can be done by setting clear goals and metrics for the model and tracking its performance over time. Some common metrics for language models include accuracy, precision, recall, and F1 score. It is also important to compare the performance of the model with the baseline and the cost of implementing and maintaining the model.
In conclusion, implementing small language models in business requires careful consideration of various factors such as model selection, continuous training, and measuring impact. By following best practices and keeping up with the latest advancements in natural language processing, businesses can leverage the potential of small language models to improve their operations and gain a competitive edge in the market.
Frequently Asked Questions
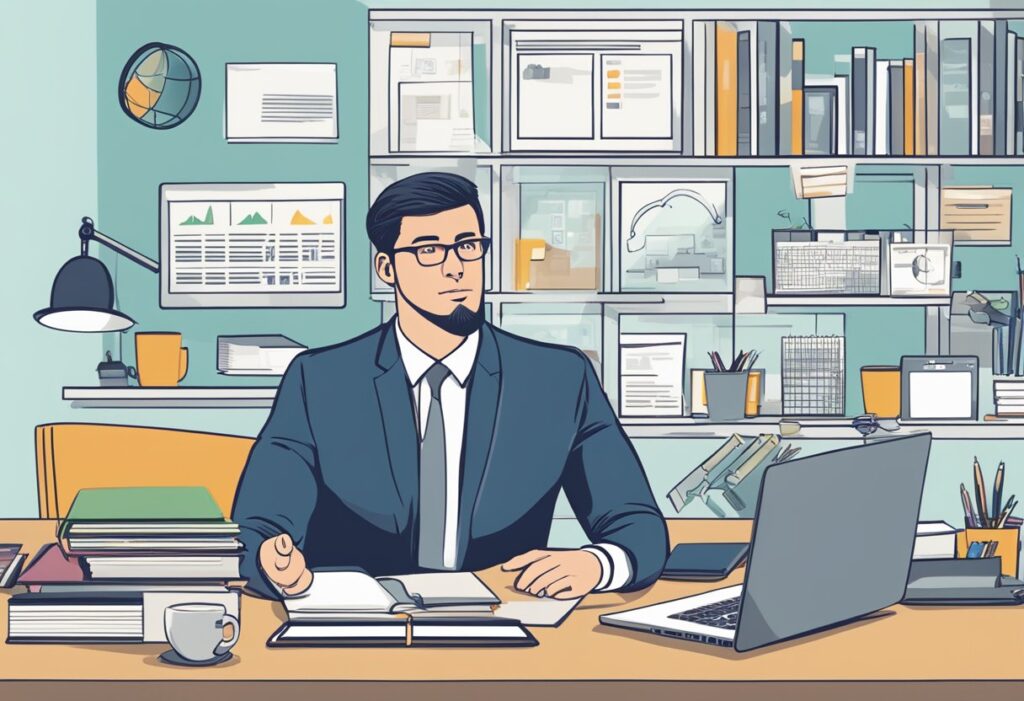
How can small language models improve customer service efficiency?
Small language models can be used to automate customer service tasks such as chatbots, voice assistants, and email responses. This can improve efficiency by reducing response times and freeing up customer service representatives to focus on more complex tasks. Small language models can also help identify common customer issues and provide quick solutions, reducing the need for human intervention.
What are the cost benefits of implementing small language models in businesses?
Small language models can help businesses save costs by reducing the need for manual labor in tasks such as data entry, content creation, and customer service. They can also help identify patterns and insights in large datasets, reducing the need for expensive data analysis software. Additionally, small language models can be trained on smaller datasets, reducing the need for large amounts of data, which can be costly to collect and store.
In what ways do small language models contribute to data analysis and insights?
Small language models can be used to analyze large datasets and identify patterns and insights that may have been missed by human analysts. They can also be used to predict future trends and outcomes based on historical data. Small language models can also help identify anomalies and outliers in datasets, making it easier to identify potential issues and opportunities.
How do small language models enhance content creation and marketing strategies?
Small language models can be used to generate content such as product descriptions, blog posts, and social media posts. This can save time and resources by reducing the need for manual content creation. Small language models can also be used to analyze social media data and identify trends and insights that can inform marketing strategies.
What are the limitations of small language models in handling complex business tasks?
Small language models may not be able to handle complex business tasks that require a deep understanding of domain-specific knowledge. They may also struggle with tasks that require reasoning or critical thinking. Small language models may also be limited by the amount and quality of training data available.
How does the integration of small language models into business workflows affect team productivity?
The integration of small language models into business workflows can improve team productivity by reducing the need for manual labor in tasks such as data entry and content creation. It can also free up team members to focus on more complex tasks that require human intervention. However, the integration of small language models may also require additional training and support for team members, which can initially reduce productivity.